Achieving Cost Reduction in Traditional ML and AI 1.0
Machine learning (ML) has become an integral part of many businesses, but the associated costs can be significant.
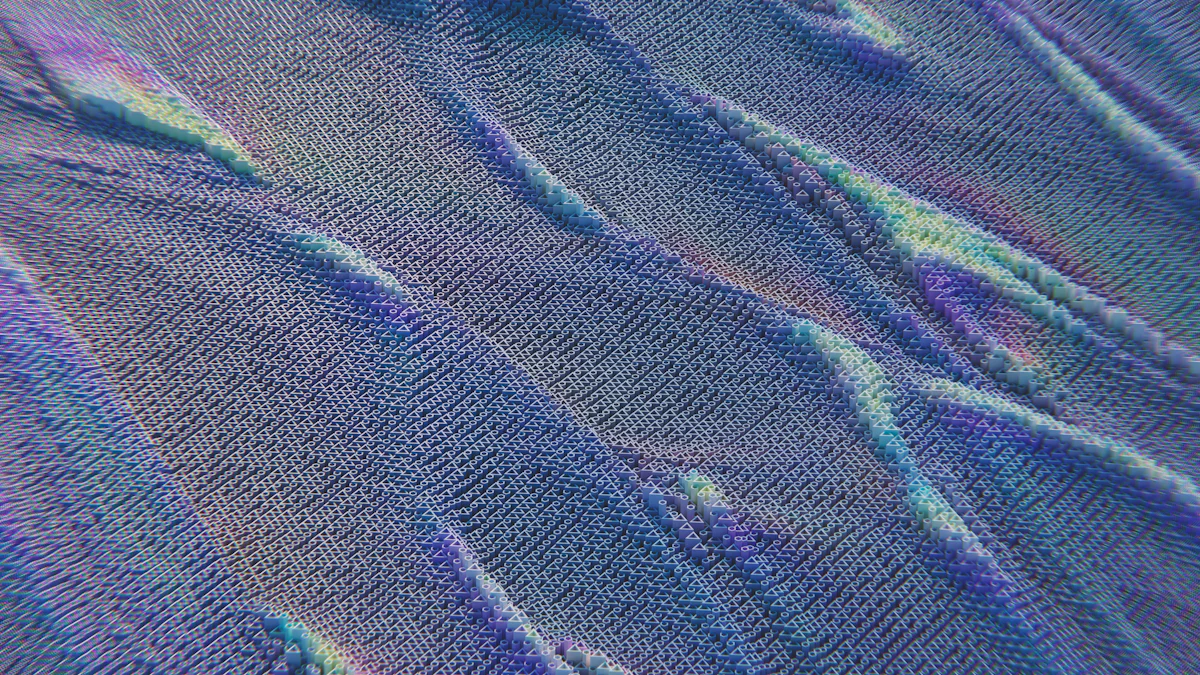
Image Source: pexels
Addressing High Costs with Traditional ML and AI 1.0
Machine learning (ML) has become an integral part of many businesses, but the associated costs can be significant. The expenses related to ML infrastructure and talent can pose challenges for organizations looking to adopt this technology. However, by implementing Traditional ML and AI 1.0, enterprises can reduce costs without compromising performance.
Traditional ML and AI 1.0 offer a practical solution to address the high costs associated with ML. This approach focuses on leveraging existing resources, optimizing processes, and upskilling existing talent rather than relying on expensive infrastructure or external resources. By adopting these strategies, enterprises can reduce expenses while still driving value through the effective use of machine learning techniques.
In the following sections, we will explore various steps that organizations can take to achieve cost reduction using Traditional ML and AI 1.0. From evaluating existing ML infrastructure to leveraging pre-trained models and open-source tools, we will provide practical insights into how businesses can optimize their machine learning practices while minimizing costs. Let's dive in!
Evaluating Existing ML Infrastructure
To achieve cost reduction with Traditional ML and AI 1.0, it is crucial to evaluate the existing ML infrastructure and identify areas for optimization. This evaluation helps in identifying inefficiencies and determining the cost-effectiveness of existing ML tools and platforms.
Identifying areas for optimization
Assessing the current ML infrastructure is the first step towards reducing costs. It involves analyzing the performance of various components, such as hardware, software, and data storage systems. By identifying bottlenecks or areas that require improvement, organizations can optimize their infrastructure to reduce unnecessary expenses.
Evaluating the cost-effectiveness of existing ML tools and platforms is equally important. While some tools offer advanced features, they might come at a higher price point. By assessing the value provided by each tool to its cost, businesses can make informed decisions about which tools are essential and which can be replaced with more cost-effective alternatives.
Streamlining data storage and processing
Data storage and processing are critical aspects of any ML workflow. Optimizing these processes can significantly impact costs. Organizations can start by assessing data storage requirements and eliminating redundant or unused data. Implementing efficient data retrieval mechanisms ensures that only relevant data is accessed, reducing storage costs.
Leveraging cloud-based solutions can also contribute to cost-effective scalability. Cloud platforms provide flexible storage options that allow businesses to scale their infrastructure based on demand. This eliminates the need for upfront investments in expensive hardware while ensuring optimal resource allocation.
By evaluating existing ML infrastructure, identifying areas for optimization, streamlining data storage and processing, and leveraging cloud-based solutions, enterprises can lay a strong foundation for achieving cost reduction with Traditional ML and AI 1.0 practices. In the next section, we will explore how organizations can further reduce development costs by leveraging pre-trained models and open-source tools.
Leveraging Pre-Trained Models and Open-Source Tools
To achieve cost reduction with Traditional ML and AI 1.0, organizations can leverage pre-trained models and open-source tools. These resources offer opportunities to reduce development costs while maintaining performance and driving value.
Reducing development costs
Utilizing pre-trained ML models is a valuable strategy to accelerate development and reduce costs. Pre-trained models are trained on large datasets by experts, making them capable of performing various tasks without extensive training from scratch. By leveraging these models, businesses can save time and resources that would otherwise be spent on training their models.
In addition to pre-trained models, open-source tools and libraries provide cost savings by offering free or low-cost alternatives to proprietary software. These tools often have active communities that contribute to their development, ensuring continuous improvement and support. By utilizing open-source tools, organizations can access various functionalities without incurring additional expenses.
Customizing pre-trained models
While pre-trained models offer significant advantages, they may not align perfectly with specific business needs. However, customization can bridge this gap while minimizing development time and costs. Organizations can adapt pre-trained models by fine-tuning them on their datasets or modifying specific layers to suit their requirements. This approach allows businesses to benefit from the efficiency of pre-trained models while tailoring them to address particular use cases.
By leveraging pre-trained models and open-source tools, enterprises can significantly reduce development costs associated with machine learning projects. The next section will explore how optimizing resource allocation further contributes to cost reduction while maximizing efficiency in ML workflows.
Optimizing Resource Allocation
Optimizing resource allocation is a crucial step in achieving cost reduction with Traditional ML and AI 1.0. By right-sizing infrastructure and implementing cost-effective hardware solutions, organizations can maximize efficiency while minimizing unnecessary expenses.
Right-sizing infrastructure
Scaling ML infrastructure based on actual needs is essential to avoid overprovisioning and reduce unnecessary costs. It involves evaluating the workload requirements and allocating resources accordingly. By accurately estimating the computational power, storage capacity, and network bandwidth needed for ML projects, businesses can optimize their infrastructure without overspending on excessive resources.
Avoiding overprovisioning also helps prevent underutilization of resources, which can lead to wasted investments. By closely monitoring resource usage and adjusting capacity as required, organizations can ensure that their infrastructure aligns with the demands of their ML workflows.
Implementing cost-effective hardware solutions
Exploring affordable hardware options designed explicitly for ML workloads is another way to optimize resource allocation. Traditional ML and AI 1.0 techniques can often be executed efficiently on less expensive hardware configurations without compromising performance.
Organizations should consider alternatives such as specialized processing units or GPU virtualization to optimize resource utilization. These solutions offer cost savings by providing efficient computation capabilities while reducing energy consumption.
By optimizing resource allocation through right-sizing infrastructure and implementing cost-effective hardware solutions, enterprises can achieve significant cost reduction in their machine learning practices. In the next section, we will explore how investing in upskilling existing talent can further reduce dependency on expensive external resources.
Investing in Upskilling Existing Talent
Investing in upskilling existing talent is a strategic approach to reduce dependency on expensive external resources and achieve cost reduction with Traditional ML and AI 1.0. By providing training opportunities and promoting cross-functional collaboration, organizations can enhance their internal capabilities while minimizing outsourcing costs.
Reducing dependency on external resources
Providing training opportunities to enhance ML skills among existing employees is a cost-effective way to build an in-house team of experts. By investing in training programs, businesses can equip their workforce with the knowledge and skills to tackle ML projects internally. This reduces the need for outsourcing expensive external talent and allows organizations more control over their ML initiatives.
Building an in-house ML team not only saves costs but also fosters a culture of innovation within the organization. With dedicated resources who understand both the business domain and machine learning techniques, enterprises can develop tailored solutions that address specific challenges effectively.
Promoting cross-functional collaboration
Encouraging knowledge sharing between data scientists and domain experts promotes cross-functional collaboration, leading to more efficient use of existing talent. By leveraging the expertise of individuals from different backgrounds, organizations can gain diverse perspectives that contribute to better problem-solving and innovative ML solutions.
Leveraging existing talent for diverse ML projects also helps maximize resource utilization. Instead of relying solely on specialized data scientists, organizations can tap into the knowledge and experience of employees from various departments who have a solid understanding of the business context and machine learning concepts.
By investing in upskilling existing talent, businesses can reduce dependency on expensive external resources while fostering internal expertise in machine learning. In the next section, we will explore how achieving cost reduction with Traditional ML and AI 1.0 can drive enterprise value by maintaining performance while reducing expenses.
Driving Cost Reduction and Value for Enterprises
By evaluating existing ML infrastructure, leveraging pre-trained models and open-source tools, optimizing resource allocation, and investing in upskilling existing talent, organizations can significantly reduce expenses without compromising performance.
Through these cost-effective ML practices, businesses can maintain high-quality results while minimizing the financial burden of ML infrastructure and talent. This enables enterprises to allocate resources more efficiently, invest in other growth areas, and ultimately create value for their stakeholders.