The Critical Role of Data Engineering in Unlocking Generative AI's Potential
Discover how CIOs can maximize AI value by investing in data quality, scalable infrastructure, and ethical practices. Learn how Data Engineering is essential for deploying AI models that drive innovation and competitive advantage.
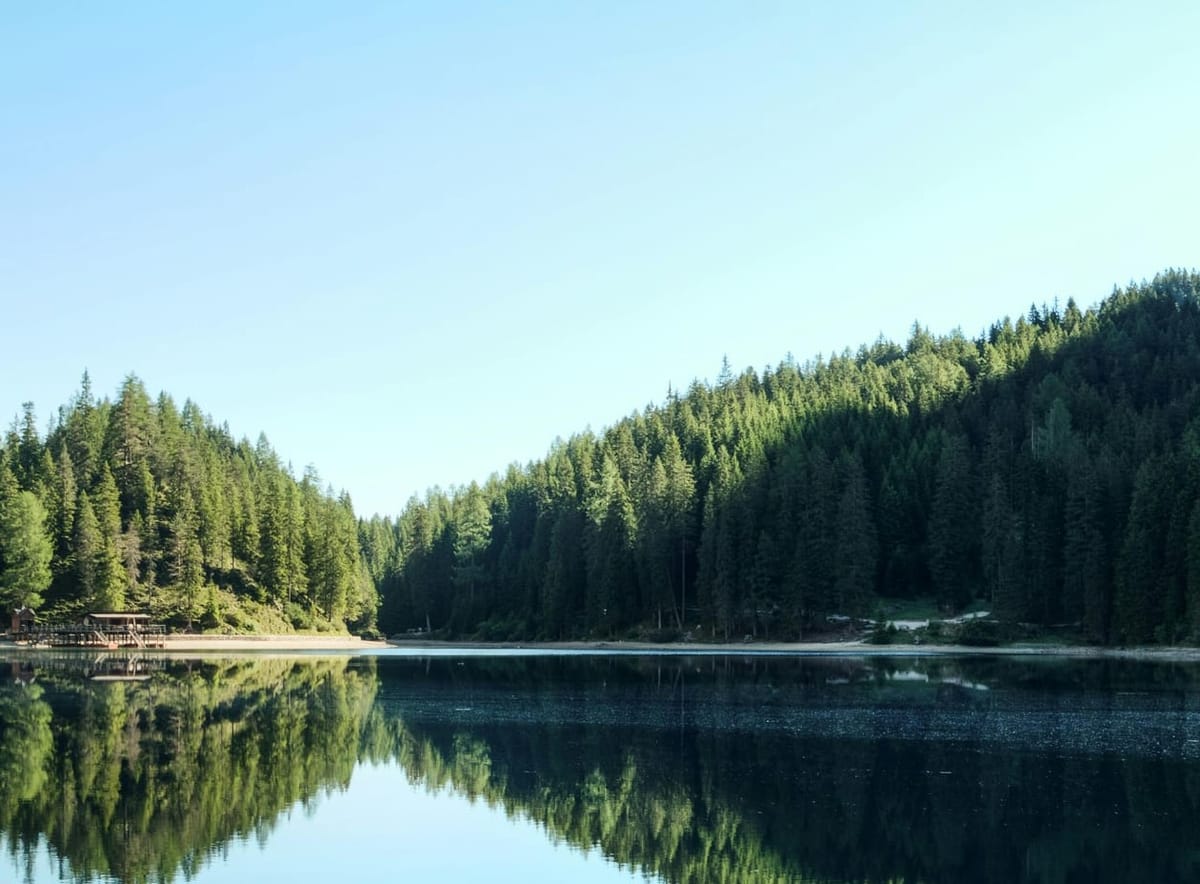
As Chief Information Officers (CIOs) and enterprise leaders navigate the rapidly evolving technology landscape, Generative Artificial Intelligence (AI) stands out as a transformative force. Generative AI promises unprecedented innovation and efficiency, from creating realistic images and coherent text to composing music and designing products. However, the true potential of these advanced models hinges on a less glamorous but equally vital discipline: Data Engineering.
Data Engineering lays the foundation upon which Generative AI models are built and operated. It encompasses designing, constructing, and maintaining the data infrastructure required to collect, store, process, and make data accessible for AI applications. Without robust Data Engineering practices, even the most sophisticated Generative AI models can falter, leading to suboptimal performance and unreliable outputs.
In this blog post, we'll explore why Data Engineering is indispensable for maximizing the value of Generative AI in an enterprise setting. We'll delve into the challenges and best practices, supported by insights from industry experts and real-world examples, to guide CIOs in strategically investing in Data Engineering for AI success.
The Symbiotic Relationship Between Data Engineering and Generative AI
Generative AI models, such as OpenAI's GPT-3 and Google's BERT, require massive amounts of high-quality data to learn patterns and generate meaningful outputs[^1^]. The effectiveness of these models is directly proportional to the quality and structure of the data on which they are trained.
Data Quality and Preprocessing
High-quality data is the lifeblood of Generative AI. Inconsistent, incomplete, or biased data can lead to models that produce erroneous or prejudiced results[^2^]. Data Engineering ensures that data is:
• Clean: Free from errors, duplicates, and inconsistencies.
• Relevant: Aligned with the AI application's specific domain and use case.
• Structured: Organized in a way that models can efficiently process and learn from.
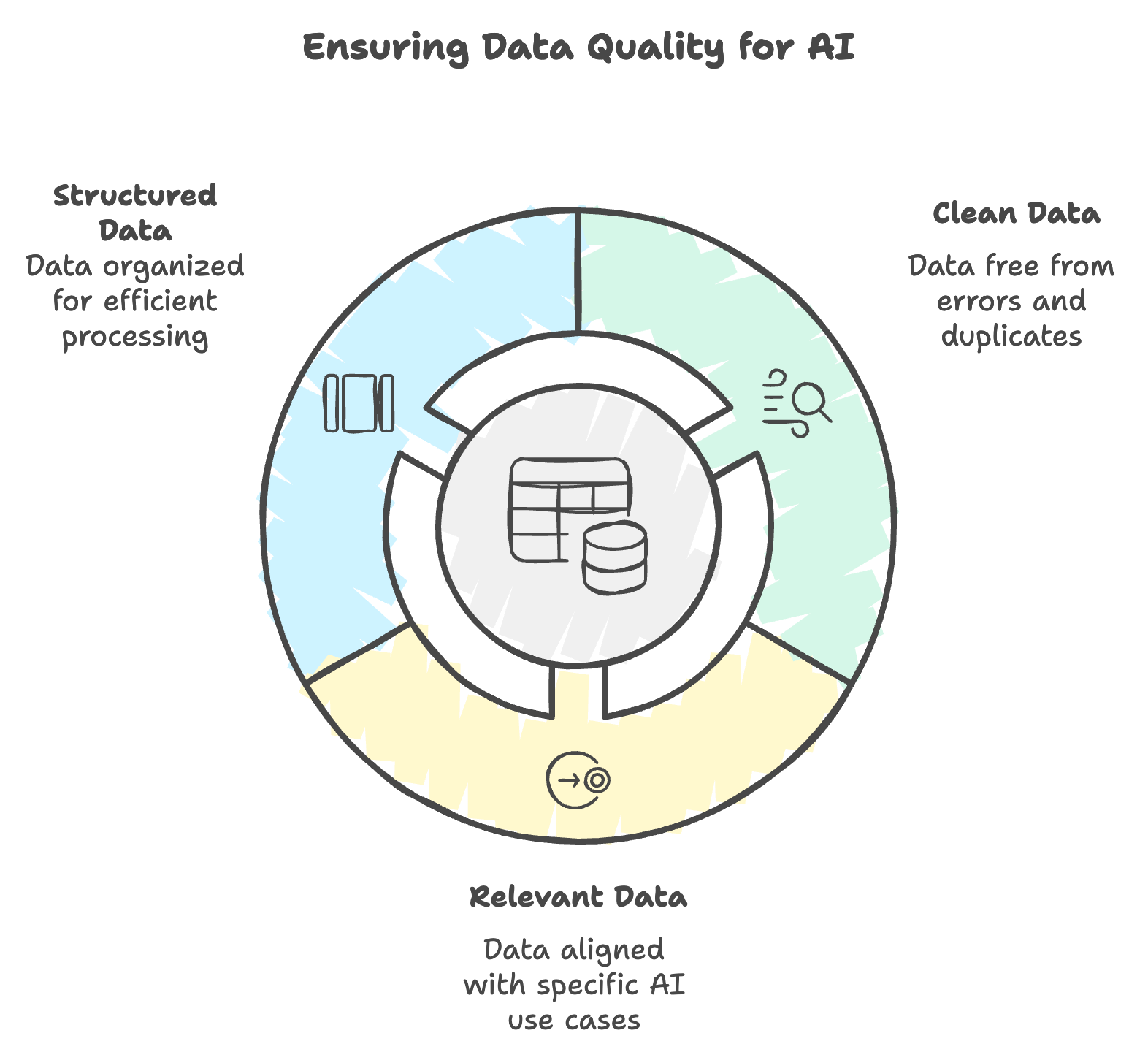
Scalability and Infrastructure
Generative AI models are computationally intensive and require scalable data infrastructures. Data Engineering provides the tools and platforms to handle large volumes of data and support real-time processing[^3^]. Technologies like distributed computing and cloud-based storage solutions enable enterprises to scale their AI initiatives without compromising performance.
Collaboration Between Teams
Effective AI implementation requires close collaboration between data engineers, data scientists, and AI researchers. Data Engineering bridges the gap by providing data that meets the specific needs of AI models[^4^]. This interdisciplinary cooperation ensures that the models are technically sound and aligned with business objectives.
Ethical Considerations and Compliance
Data privacy, security, and compliance are paramount, especially with GDPR and CCPA [^5^] regulations. Data Engineering practices help enforce data governance policies, ensuring that data used for AI is compliant with legal standards and ethical norms.
Key Challenges and Solutions in Data Engineering for Generative AI
Challenge 1: Ensuring Data Quality and Consistency
Solution: Implement robust data validation frameworks and anomaly detection systems. Tools like TensorFlow Data Validation can automate the detection of anomalies and inconsistencies in data[^6^].
Challenge 2: Scaling Data Infrastructure
Solution: Leverage cloud-based platforms and distributed systems such as Apache Spark and Kubernetes. These technologies facilitate the processing of large datasets and support the computational demands of Generative AI[^7^].
Challenge 3: Data Privacy and Security
Solution: Adopt privacy-preserving techniques like differential privacy and federated learning. These methods enable models to learn from data without exposing sensitive information[^8^].
Challenge 4: Mitigating Bias and Ensuring Fairness
Solution: Utilize bias detection algorithms and fairness-aware machine learning practices. Continuous monitoring and validation can help identify and correct biases in data and models[^9^].
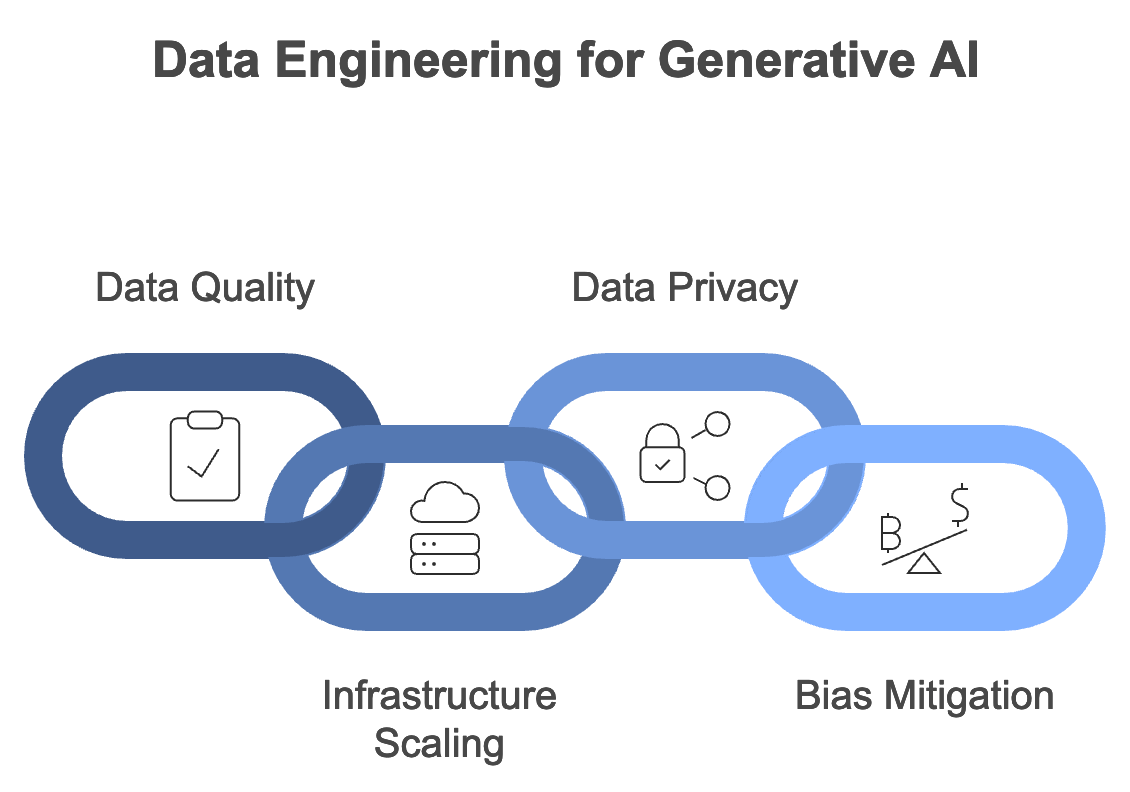
Strategic Recommendations for CIOs
1. Invest in Data Engineering Talent and Training: Building a skilled Data Engineering team is crucial. Continuous learning opportunities ensure your team stays updated with the latest tools and best practices.
2. Foster Cross-Functional Collaboration: Encourage collaboration between data engineers, data scientists, and business units to align AI initiatives with organizational goals.
3. Implement Robust Data Governance: Develop clear policies and frameworks for data management, privacy, and compliance. Tools that track data lineage and versioning can enhance transparency.
4. Adopt Scalable Technologies: Utilize cloud services and scalable architectures to handle growing data and computational requirements.
5. Prioritize Ethical AI Practices: Integrate ethical considerations into data handling and AI model development to build trust and comply with regulations.
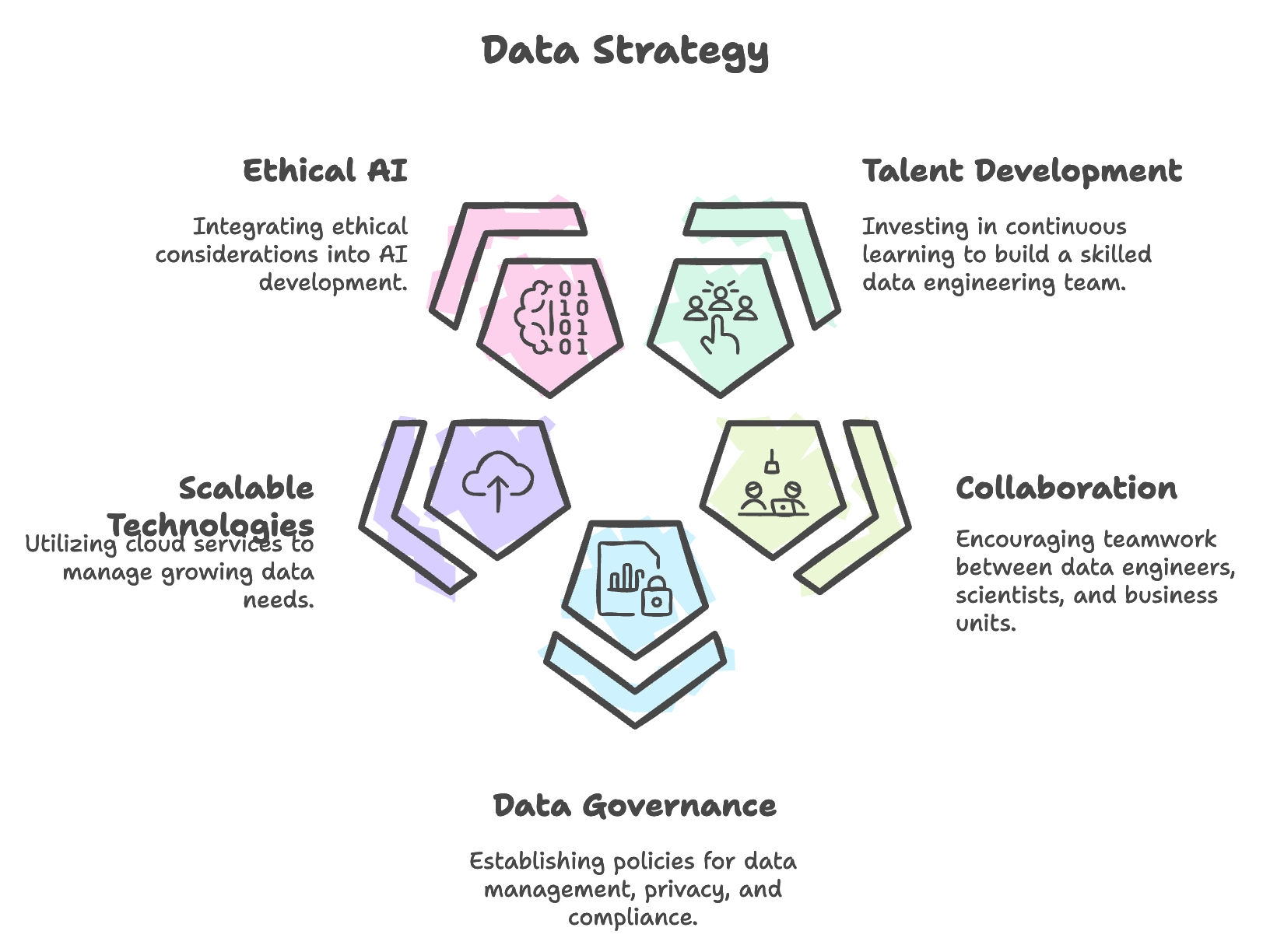
Real-World Impact: Case Studies
Healthcare Innovation
A leading healthcare provider leveraged Data Engineering to preprocess and securely integrate vast patient data. By ensuring data quality and compliance, they successfully deployed a Generative AI model that predicts patient readmissions, improving care and reducing costs[^10^].
Financial Services Enhancement
A multinational bank used advanced Data Engineering techniques to consolidate and cleanse transactional data. This enabled the deployment of AI models for fraud detection and personalized financial advice, enhancing security and customer satisfaction[^11^].
Conclusion
For enterprises aiming to harness the transformative power of Generative AI, investing in robust Data Engineering practices is not just beneficial—it's imperative. As CIOs and technology leaders, prioritizing Data Engineering will set the stage for AI initiatives that are scalable, ethical, and aligned with business objectives.
By addressing challenges in data quality, infrastructure scalability, collaboration, and ethical compliance, organizations can unlock the full potential of Generative AI. This strategic focus will drive innovation, operational efficiency, and competitive advantage in the rapidly evolving digital landscape.
Sources
[^1^]: Brown, T. B., Mann, B., Ryder, N., et al. (2020). Language Models are Few-Shot Learners. arXiv:2005.14165
[^2^]: Suresh, H., & Guttag, J. V. (2021). A Framework for Understanding Sources of Harm throughout the Machine Learning Life Cycle. arXiv:1901.10002
[^3^]: Zaharia, M., Chowdhury, M., Franklin, M. J., Shenker, S., & Stoica, I. (2010). Spark: Cluster Computing with Working Sets. Proceedings of the 2nd USENIX Conference on Hot Topics in Cloud Computing.
[^4^]: Saltz, J. S., & Shamshurin, I. (2016). Big Data Team Process Methodology: A Literature Review and Identifying Key Factors for a Project's Success. 2016 IEEE International Conference on Big Data.
[^5^]: Voigt, P., & Von dem Bussche, A. (2017). The EU General Data Protection Regulation (GDPR). Springer International Publishing.
[^6^]: TensorFlow. (n.d.). TensorFlow Data Validation. https://www.tensorflow.org/tfx/data_validation
[^7^]: Apache Software Foundation. (n.d.). Apache Spark™ - Unified Analytics Engine for Large-Scale Data Processing. https://spark.apache.org/
[^8^]: Dwork, C., & Roth, A. (2014). The Algorithmic Foundations of Differential Privacy. Foundations and Trends® in Theoretical Computer Science.
[^9^]: IBM Research. (n.d.). AI Fairness 360. https://aif360.mybluemix.net/
[^10^]: Esteva, A., Robicquet, A., Ramsundar, B., et al. (2019). A Guide to Deep Learning in Healthcare. Nature Medicine, 25(1), 24–29.
[^11^]: Bhatia, A., Jenssen, R., & Martens, J. (2021). Machine Learning in Finance: The Case for AI-driven Credit Scoring. Journal of Financial Regulation and Compliance.