The Hidden Traps of "Business Value" in Data-Driven Decisions:
Overemphasizing “business value”—often a vague and subjective term—in data decisions can mislead organizations. Instead, leaders should prioritize projects based on criticality, deployability, and core needs.
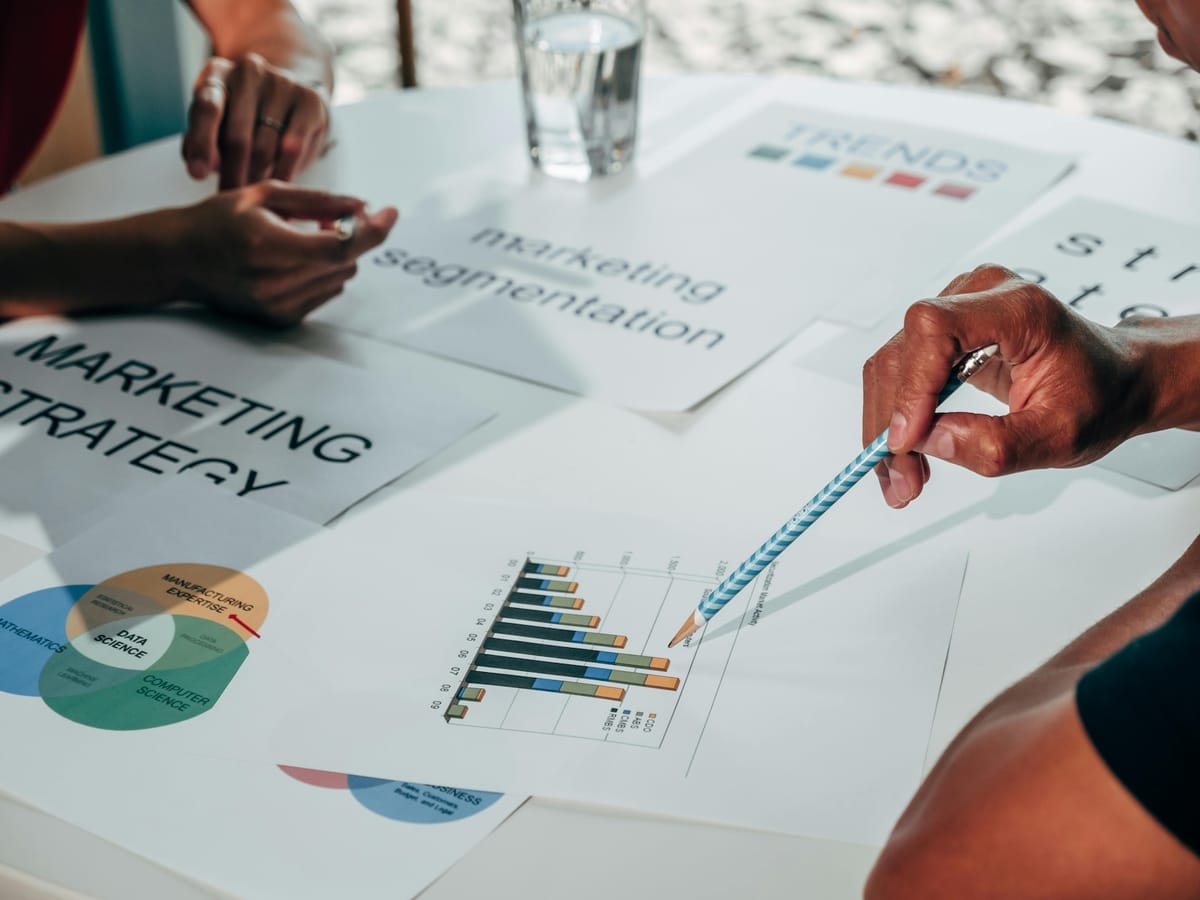
A Critical Guide for CIOs and Leaders
In today's data-saturated business landscape, organizations are under immense pressure to leverage data for competitive advantage. CIOs and other leaders are tasked with extracting tangible "business value" from data-driven initiatives. While pursuing business value is a logical objective, this concept's overemphasis—or misinterpretation—can lead to significant pitfalls.
This article delves into the common traps associated with using "business value" as the sole driver for data-driven decisions. We aim to provide a critical thinking framework for leaders to navigate these challenges effectively, ensuring that data initiatives contribute meaningfully to long-term organizational success.
The Subjectivity and Ambiguity of "Business Value"
Defining the Indefinable
At first glance, "business value" seems straightforward: initiatives should contribute positively to the organization's bottom line. However, the term is often a vague catch-all, lacking a consistent, universally understood definition. Much like buzzwords such as "innovation" or "digital transformation," its meaning can vary widely across different contexts and stakeholders.
Diverse Interpretations Across Departments
Within an organization, different departments may have contrasting views on what constitutes business value:
• Marketing might prioritize brand awareness and customer engagement.
• R&D could focus on innovation and product development.
• Supply Chain may emphasize efficiency and cost reduction.
This subjectivity can lead to misaligned expectations and priorities, causing friction when allocating resources or defining project objectives. With a shared understanding, initiatives can avoid becoming siloed, undermining the organization's overarching goals.
The Short-Sightedness of Immediate Financial Returns
The Allure of Quick Wins
In an environment driven by quarterly earnings and shareholder expectations, there's a solid temptation to prioritize projects that promise immediate financial returns. While these "quick wins" can provide a temporary boost, they may not contribute to sustainable growth or competitive advantage.
Strategic Investments Require Patience
Consider industries like pharmaceuticals or aerospace, where R&D investments are substantial and long-term. The payoff from these endeavors is brief, but they're crucial for future success and market leadership. Similarly, data initiatives should be viewed as strategic investments:
• Building Data Infrastructure: Establishing robust data pipelines and storage solutions may not show immediate ROI, but they are essential for scalability.
• Developing Advanced Analytics Capabilities: Investing in machine learning and AI can unlock insights that drive innovation over time.
• Fostering a Data-Driven Culture: Cultivating organizational skills and mindsets enhances decision-making and agility.
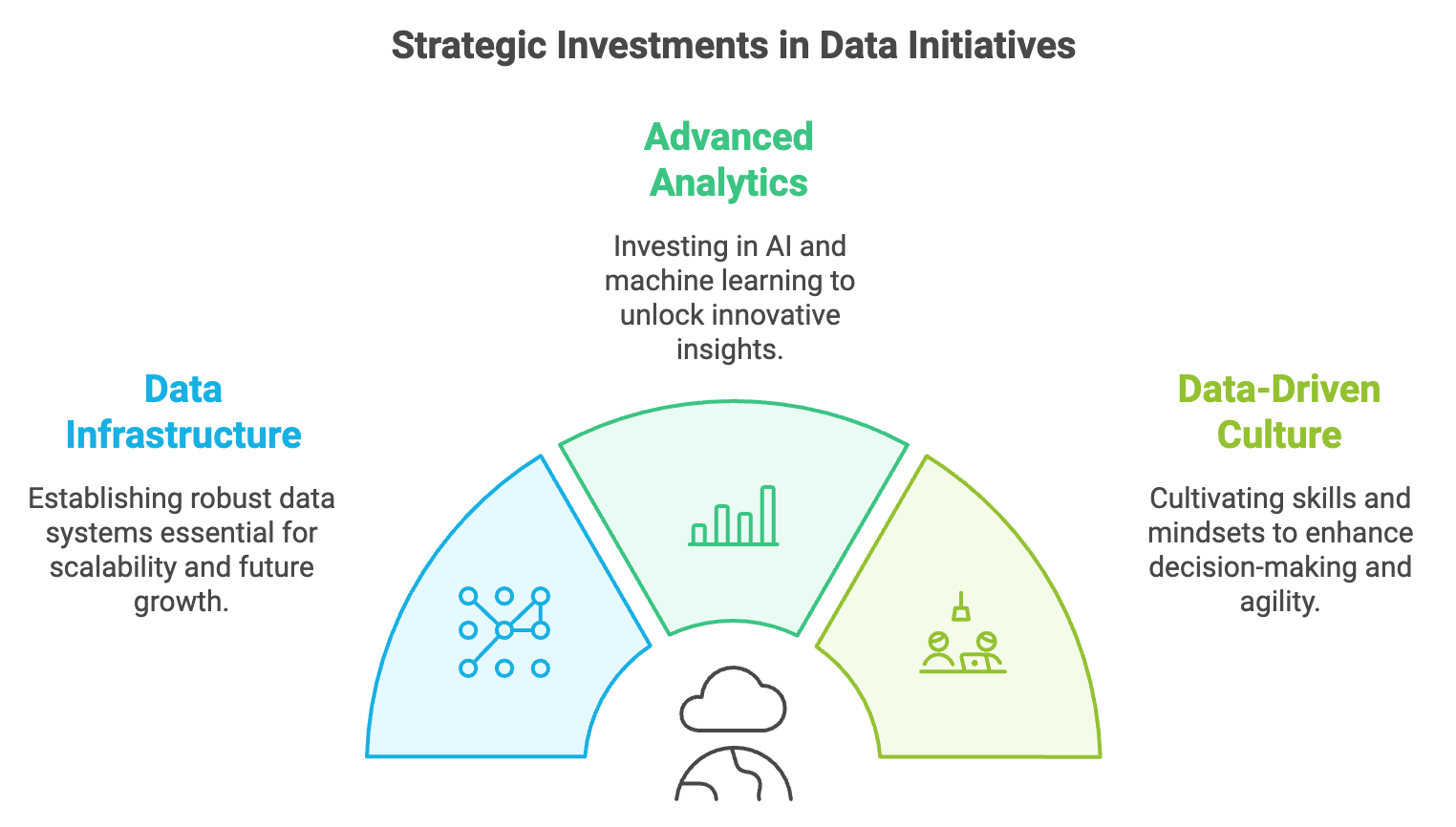
Focusing solely on short-term financial metrics can stifle these foundational efforts, leaving organizations ill-prepared for future challenges.
The Pitfalls of Using "Business Value" as a Bargaining Chip
Negotiation at the Expense of Strategy
When budget constraints tighten, "business value" often becomes a bargaining tool to justify cuts or reallocate resources. Projects that don't demonstrate immediate value are postponed or canceled, regardless of their long-term importance.
Undermining Data Initiatives
This approach can have detrimental effects:
• Erosion of Data Quality: Underfunded data initiatives may lead to poor data quality, which can impact all downstream applications.
• Loss of Talent: Inconsistent support for data projects can demotivate skilled professionals, leading to attrition.
• Missed Opportunities: The organization may lag in effectively adopting new technologies or methodologies that competitors leverage.
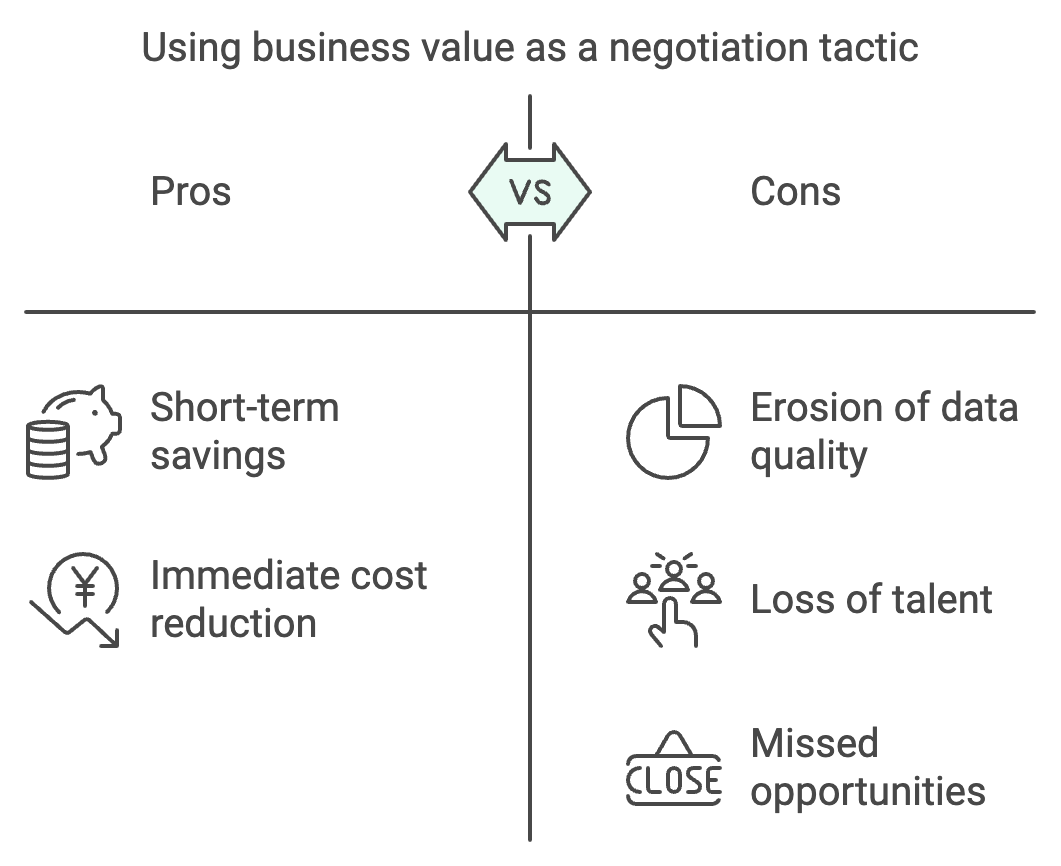
Using business value as a negotiation tactic undermines the strategic potential of data-driven decision-making, potentially causing more harm than the short-term savings justify.
Alternative Frameworks for Prioritizing Data Projects
To avoid these traps, leaders should consider more holistic frameworks that account for both immediate needs and long-term goals.
1. Criticality and Deployability
Assessing Criticality
Evaluate projects based on their importance to the organization's longevity and strategic objectives:
• Regulatory Compliance: Projects necessary to meet legal requirements.
• Market Differentiation: Initiatives that offer a competitive edge.
• Operational Efficiency: Efforts that streamline core processes.
Evaluating Deployability
Consider the feasibility and complexity of implementing the project:
• Resource Availability: Do you have the necessary talent and technology?
• Technical Complexity: What are the potential risks and challenges?
• Timeline Constraints: Can the project be delivered within a reasonable timeframe?
Prioritization Matrix
By mapping projects on a matrix of criticality vs. deployability, organizations can:
• Prioritize High-Impact, Feasible Projects: Focus on critical and deployable initiatives.
• Plan for Complex but Critical Projects: Allocate resources for essential projects that may take longer to implement.
• Defer Low-Criticality Projects: Postpone or rethink initiatives that offer minimal strategic value.
2. Core, Common, and Local Needs
Conducting a Forensic Analysis
Engage with stakeholders across all levels and departments to identify pain points and challenges:
• Interviews and Surveys: Gather qualitative and quantitative data on operational issues.
• Cross-functional workshops: Facilitate discussions to uncover hidden dependencies and synergies.
Categorizing Challenges
• Core Issues: Problems affecting the entire organization (e.g., data silos, inconsistent metrics).
• Common Issues: Challenges faced by multiple departments (e.g., inefficient reporting tools).
• Local Issues: Department-specific or team-specific problems.
Strategic Focus
• Address Core Issues First: Solutions here will have the broadest impact.
• Tackle Common Issues Next: Improves efficiency across several areas.
• Local Issues as Tailored Solutions: Customize approaches for specific needs without diverting excessive resources.
This methodology ensures efforts are concentrated where they matter most, enhancing overall organizational effectiveness.
Embracing a Holistic Approach to Data Value
Aligning with Organizational Strategy
Data initiatives should be tightly integrated with the organization's long-term strategy:
• Vision and Mission Compatibility: Ensure projects support overarching goals.
• Adaptive Roadmaps: Create flexible plans that can evolve with changing business landscapes.
Investing in Foundational Capabilities
Building a solid data foundation is essential:
• Data Governance: Implement policies and standards for data quality and security.
• Scalable Infrastructure: Leverage cloud technologies and modular architectures.
• Skill Development: Invest in training and hiring to build a competent data team.
Leveraging Advanced Technologies
Utilize cutting-edge technologies like Generative AI and advanced analytics to unlock new possibilities:
• Automated Insights: Accelerate decision-making with AI-driven analysis.
• Personalized Experiences: Enhance customer engagement through tailored offerings.
• Predictive Modeling: Anticipate trends and adjust strategies proactively.
Conclusion
The quest for "business value" in data-driven decisions is fraught with potential traps that can hinder an organization's growth and adaptability. Leaders can steer their organizations toward more meaningful outcomes by recognizing the term's subjectivity, avoiding the short-sighted focus on immediate returns, and not using it as a mere bargaining chip.
Adopting alternative frameworks like Criticality and Deployability and addressing Core, Common, and Local Needs provides a more nuanced approach to prioritizing data projects. This holistic perspective ensures that data initiatives are aligned with strategic objectives, feasible to implement, and impactful across the organization.
Next Steps for Leaders:
• Re-evaluate Your Definition of Business Value: Foster a shared understanding across your organization.
• Assess Your Current Data Initiatives: Use the frameworks provided to prioritize effectively.
• Invest in Foundational Capabilities: Strengthen your data infrastructure and talent pool.
• Explore Advanced Technologies: Consider how AI and analytics can enhance your data strategy.
• Engage with Experts: Seek external guidance to bolster your internal efforts.
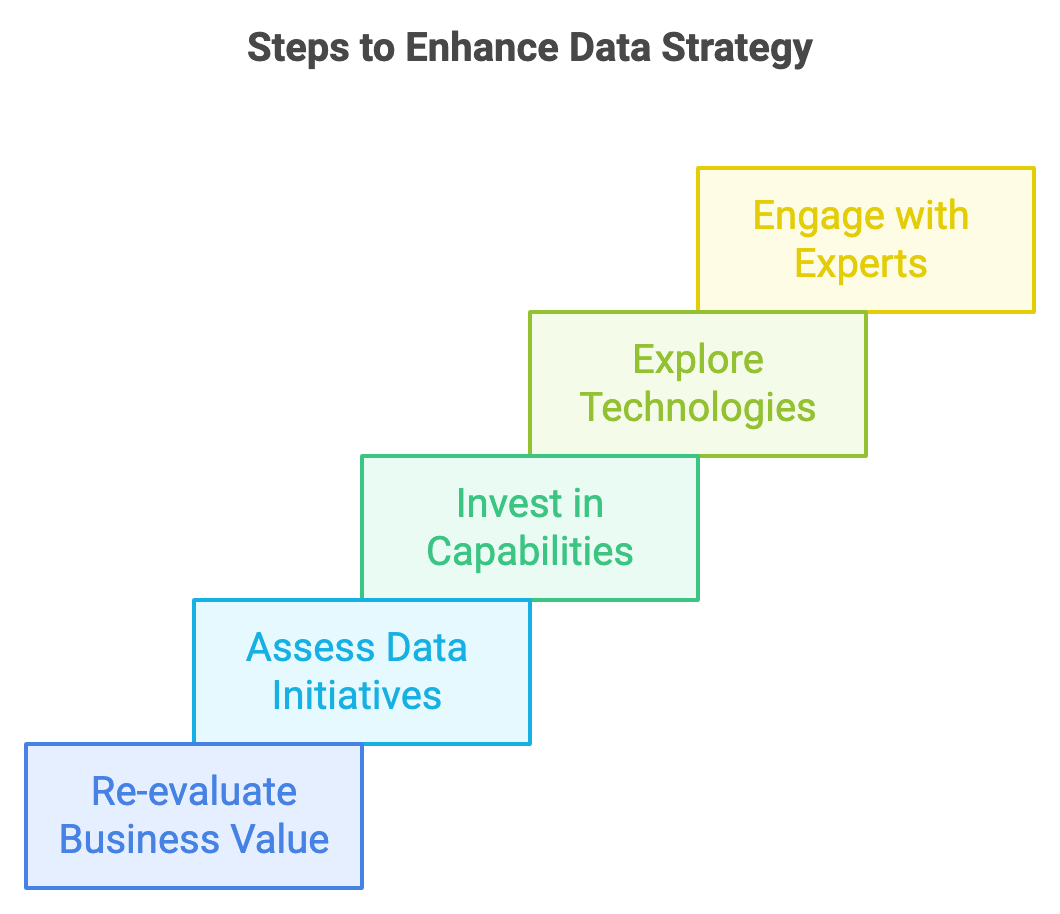
By approaching data-driven decisions with critical thinking and strategic intent, CIOs and leaders can avoid common pitfalls and unlock transformative value for their organizations.